Will The ‘Last Mile’ Of Inflation Be the Hardest? Three Reasons Why It May Not.
A place to organize my thoughts, debut my new inflation density app, put code somewhere, and outline some things I'm watching on the inflation front as we go into the end of 2023.
Against the backdrop of recent disinflation hangs a single question: is the last mile going to be the hardest? We’ve been able to bring down inflation significantly without unemployment going up, so far. But, with inflation still elevated, is that going to be true going forward, and will it become less true as it continues?
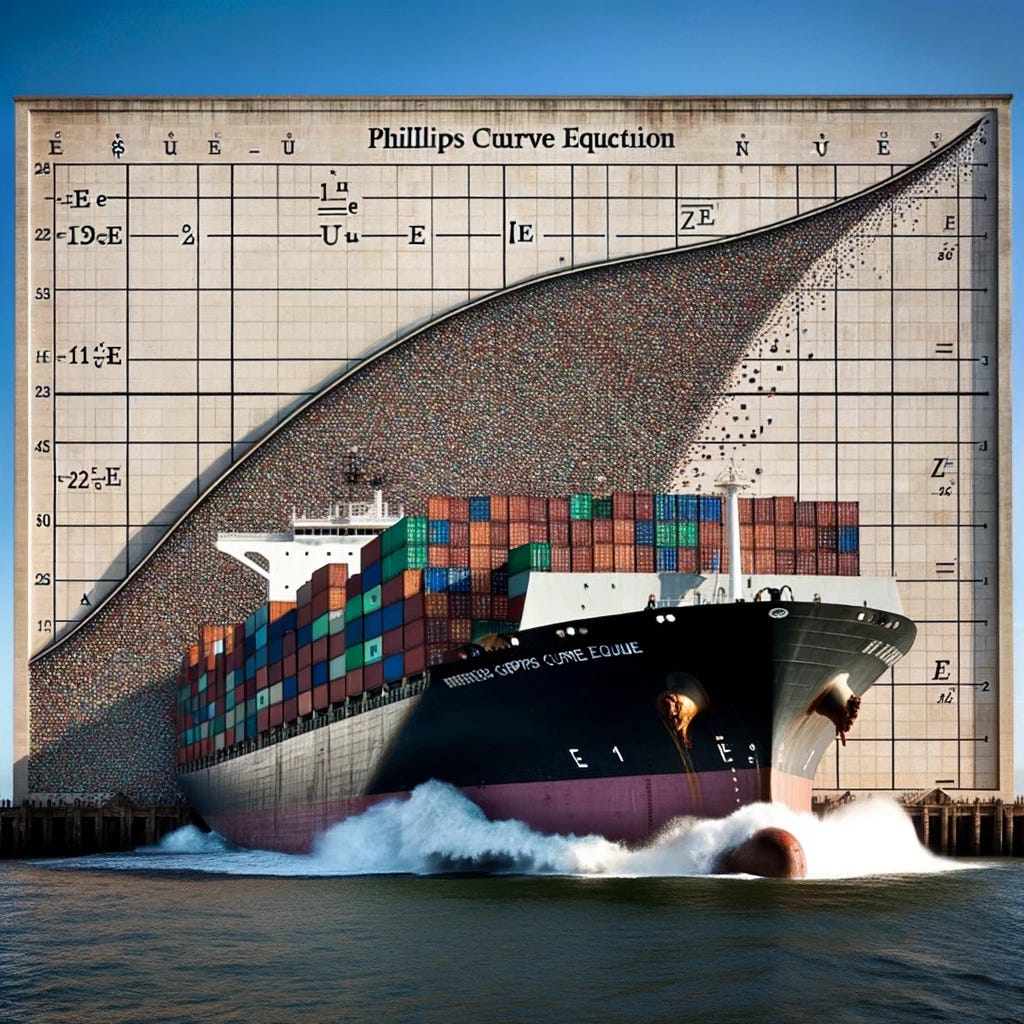
Here’s an optimistic case from Chicago Fed President Austan Goolsbee (see also Odd Lots) for a “golden path” in which inflation can come down easily, which leans on the resolution of the supply shock we’ve already seen, the anchoring of future expectations, and further disinflation in the housing market. I agree these are all positive signs.
But I want to take the opposite approach here. Let’s try to develop the best case that the last mile will, in fact, be the hardest. What form would such an argument take? I’m going to analyze three here:
Higher underlying inflation is being masked by the tails of the distribution.
Disinflation is driven by categories that aren’t sensitive to demand.
We’ve been on a nonlinear Phillips Curve, and are approaching the flat region with a higher sacrifice ratio.
There are more, but I want to start with these three for this post. Putting them under an empirical spotlight, they appear pretty thin. That’s not to say they couldn’t be right - we don’t know. But, as of right now, there’s no reason in the data to just assume they are correct. Looking at these, if anything, makes the case that supply shocks have been the driver even stronger. Let’s take each of these in turn. (Code for all the following is here.)
Story 1: Distribution
The first argument would be that it's the quirks of the distribution masking how persistent underlying inflation is. This could be the case if inflation has mostly come down from specific categories, or solely from a subset of prices becoming very negative. But this is not true in the data. The Atlanta Fed has an Underlying Inflation Dashboard of year over year changes in nine inflation metrics, which generally look at measures of the central tendency of the distribution of price changes, like the median change or the change excluding the top and bottom 8 percent of weighted changes. They’ve all come down during the past year. There’s been some reversion in recent months, but the overall arc is clear.
I’d prefer to just look at the distribution itself. There wasn’t a good dashboard, either at a regional Fed or elsewhere, to view changes in the distribution of CPI and PCE prices over different time periods and compare them, so I went ahead and made one myself and stuck it at Mike Konczal dot com here. (I plan on updating it each month, so bookmark it for the future!)
Let’s show the distribution of 6-month price changes for now, a year ago, and 4 years ago to 2019, excluding the top and bottom 3 percent. Let’s do that for 179 low-level, non-overlapping prices in the CPI (PCE, running colder, gives us the same story). It looks like this:
As we can see, the central part of the distribution of price changes has moved over dramatically in the past 12 months. Compared to 2019, it is hotter, but the negative categories aren’t significantly more negative and the central part of the distribution is lining up nicely. The disinflation we’ve experienced has been broad.
Also, since we’re pulling PCE changes from the underlying NIPA tables, we can easily compare now to the 1970s. Larry Summers argued on X this moment might be like 1976; however a thing that stands out with that comparison (and other late 1970s dates) is how little of the 1970s distribution is at or below 0 percent.
That, to me, is where expectations begin to play a role, that every industry is assuming positive price increases. We don’t see that now.
Story 2: Demand Inertial Categories
The second story would argue that disinflation is from easy, low-hanging, supply-constrained categories, and we haven’t made progress on any ‘high-hanging’ inflation, or on the categories of consumer spending where prices are most sensitive to demand conditions. We can create that high-hanging basket however. We create a Phillips Curve, a measure of aggregate supply that traces inflation into movements in output, here measured by unemployment, for each item in the consumer basket. We’ll go with CPI here; but, as I showed using different methods in this paper, the general results hold for PCE too.
First, we take the most demand-inertial specification of the accelerationist Phillips Curve, where expected inflation is just what it was last month:
We regress this equation on each item of 179 individual, non-overlapping items in the CPI from 2000 onward. Then we only select items that have a negative estimate on the second beta estimate, the unemployment gap, with a t-statistic lower than negative -2. Here are those items:
Housing is a big component of this, and we know we’ve made progress on housing and will continue to do so. But, on the first pass, the rest seems plausible enough to proceed. From pet food to auto repair to daycare to restaurants (‘full service meals and snacks’1), these are categories I can imagine people spending more money on when they feel confident in their ability to earn and spend nominal dollars.
Excluding housing, this is about 8 percent of the CPI basket, not enough to broadly generalize but significant enough to look at a trend. Have we made any progress on this? Let’s wrap these categories up into their own index and graph it:
Over the past year this too has declined. Now last month it saw a reversal. (So did other alternative measures, like median CPI.) So it is something to continue to watch. But the disinflation so far in 2023 has definitely come just as much from categories we’d understand to be inertial as it has from everything else.
Story 3: Nonlinear Phillips Curve
The third argument is that the Phillips Curve is nonlinear and convex in this range, like an U. Or, that a small initial decrease in demand (or increase in unemployment) could lead to a major drop in inflation (like moving in from the outer part of the letter U), but that this becomes harder with time as the curve becomes flatter (the center of the letter U), so you need larger drops in demand to get smaller decreases in inflation.
On the surface this seems implausible, as the disinflation is just too large given unemployment. One representative pre-pandemic estimate of the slope of a nonlinear Phillips Curve had it going from -0.14 in the flat range to -0.42 in the convex range (Hooper, Mishkin, Sufi, 2019). That’s not going to do it now; year over year core CPI fell 2.5 percent, from 6.6 to 4.1, while unemployment increased 0.3, from 3.5 to 3.8 percent. That would mean a Phillips Curve slope of -8.3.
Job Openings
During the recovery several papers have come out arguing that the Phillips Curve is nonlinear if you use job openings for vacancies and divided that by unemployment (so v/u) 2 instead of unemployment as the level of overall demand (Crust, Lansing, and Petrosky-Nadeau, 2023; Benigno and Eggertsson, 2023, Cecchetti et al 2023). There is some sense in this, given the moving unemployment rate hasn’t felt like the right measure of labor demand in this recovery. (I think there are flaws in using job openings, and we’ll move this to quits in a second.)
So how does that look? Let’s do 2000- 3-month core PCE inflation versus job-openings over unemployment, taking a regression with a squared v/u ratio:
The problem is that inflation has fallen much faster than any nonlinear or ‘steep’ (e.g. Figura and Waller, 2022) v/u relationship. It has slide entirely off the path.
Quits
We’re trying to make the best version of the argument, so what if we look at quits instead? Looking at estimates, “quits + quits²” has the best R². Here we could argue we’re on a flat part of the Phillips Curve.
But this brings us to the question of 'flatness.’ Many jump to say that it means a severe sacrifice ratio is in play. But I think it’s worthwhile to go back to why people were discussing nonlinear Phillips Curve in the 2010s: the ‘missing disinflation’ (e.g. Trabandt and Linde, 2019) with inflation not falling with unemployment above 8 percent from 2009-2012. (Remember that?) For whatever reasons, and there are many proposed, inflation stayed below target across a wide range of labor settings.
Just looking at the pre-2020 data, does this look like we need to drag the quits rate down?
There are other arguments too. There still remains a high level of nominal spending, though corporate margins are shrinking and we could see some big productivity numbers. But the most obvious reasons we would be in trouble are weaker than you might think.
As Matt Klein at the Overshoot describes “The cost of a sit-down restaurant meal captures the entire economy in a microcosm. Workers cook meals, take orders, and clean using a mix of durable equipment and perishable ingredients. The owner has to rent a venue with a kitchen and comply with whatever local regulations and mandates are imposed by the authorities. And since most restaurant customers consider dining out to be something of a luxury, their willingness to spend (and tip) is going to be sensitive to their own financial situation and prospects.
Thus, despite being a tiny component of the broader price index, there is a remarkably tight relationship between changes in the price index for ‘full service meals and snacks’ and the underlying measure of inflation preferred by Fed officials.”
Is it v/u or u/v? Search model theorists use v/u; JOLTS reports u/v. As my friend and labor economist David Wiczer notes: “V/U has a nice linguistic analog: higher is ‘tighter.’ Also, in the baseline version of these models, the main decision maker is the firm deciding to open a new job or not and unemployed searchers are pretty passive.”
Really good work, thanks!